In Today’s business and technology, data has become one of the crucial elements. Consequently, data analytics is becoming more critical than ever. We might have come across data analytics in our daily life. For example, Google search engine uses data analytics to provide us with all relevant information on a specific topic. This blog will explore what is data, understand data analysis processes and touch on different types of analytics.
Data vs Information vs Knowledge
The terms “data”, “information”, and “knowledge” are often used interchangeably. But in reality, all of them are very different. Data refers to the collection of raw, unstructured, and unorganised facts. When those facts are structured, processed, and interpreted, it’s known as information. On the other hand, a collection of information that’s structured and organised in a way that’s easy to understand is called knowledge.
Now that we have a clear definition of data, information, and knowledge. Let’s dive into data analytics.
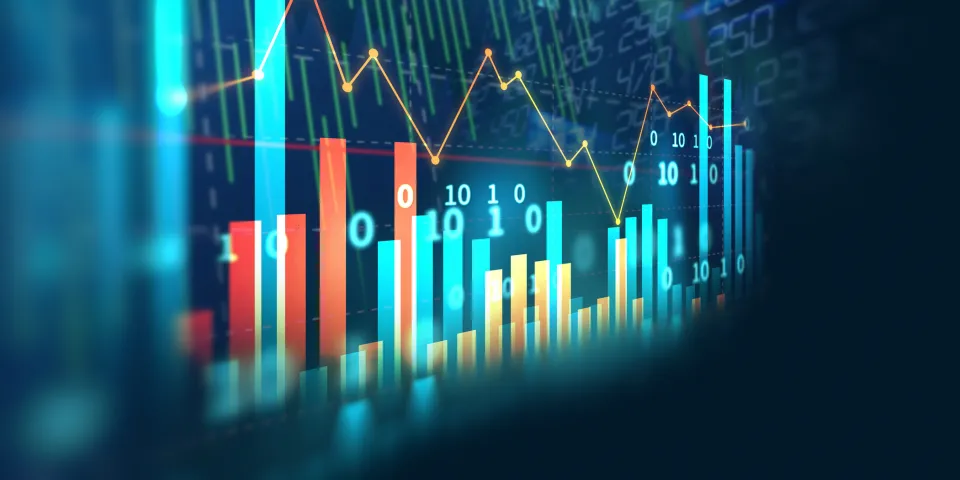
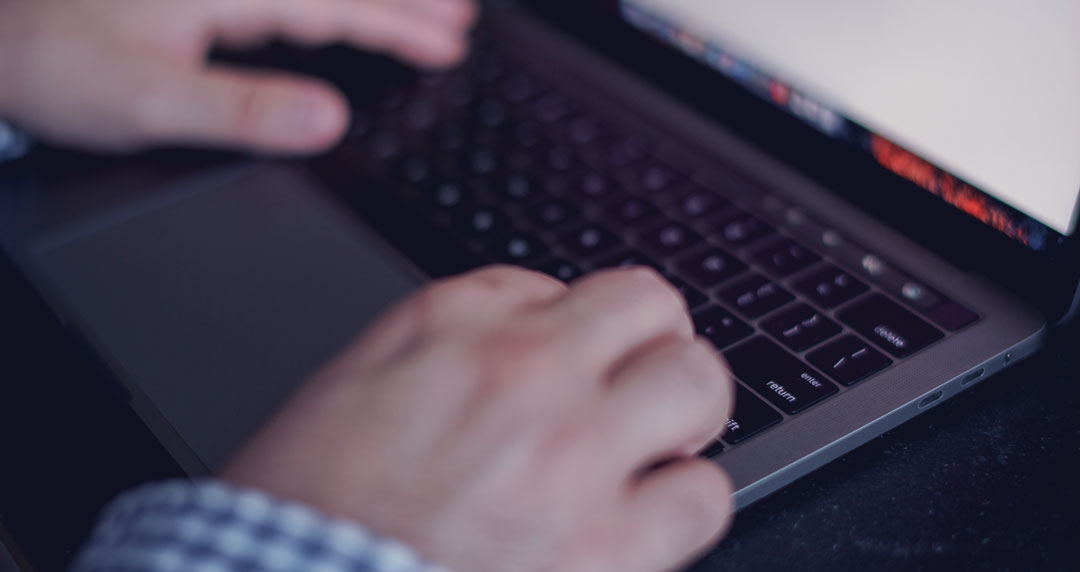
Data Analytics
How can we process raw data to gain insights from it? Data analytics is the process of analysing raw data to extract useful information and knowledge. It is essential to businesses because it can help them to optimise performance, increase revenue, drive better decisions, solve complex problems, and so on.
There are 5 essential steps to performing data analysis:
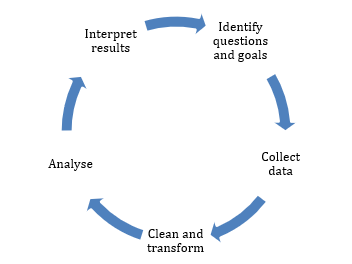
1. Define questions & goals
The first step in data analysis is to identify the questions and goals. Asking practical questions will help us better understand what we’re trying to achieve. The questions could be: What problems are we trying to solve? What are the expected outcomes of the analysis?
2. Collect data
The second step in the process is to collect data. In this stage, we identify the data sources and how we will use them in our analysis.
3. Clean and transform
Once we have collected the required data, we will prepare the data for analysis. This involves checking for invalid/incorrect data points, missing data, outliers, removing duplicates, normalising data and so on. Although all steps are important, we need to put extra care into this stage, and it is also the most time-consuming step. The data we get is now always clean and full of noise. If we don’t clean the data properly, this will lead to inaccurate and misleading information at the end of this analysis.
4. Analyse
At this stage, we can start examining our data to identify trends, patterns, correlations or make predictions that can help us answer the questions we set in the initial stage. Various types of data analysis methods are available, such as descriptive, diagnostic, predictive, and prescriptive. The kind of analysis we utilise will depend on the questions and goals defined in the initial stage.
5. Interpret results
Last but not least, it’s time to interpret our results. This phase is where we conclude what we’ve learned from the analysis and determine the best course of action based on the findings. We can show our analysis results through visualisations.
Types of data analysis
The kind of insights we get will depend on the type of analysis we perform. There are 4 main types of data analytics:
-
Descriptive Analysis
As the name suggests, the descriptive analysis looks at what has happened in the business. It simply describes what happened and doesn’t provide information on what caused it. For example, it shows an organisation’s monthly total sales for the year 2021.
-
Diagnostic Analysis
Whilst descriptive analysis only describes what has happened, diagnostic analytics seeks to understand why something happened. Its main goal is to identify and respond to outliers in our data. For example, suppose our descriptive analysis shows there is a 15% drop in sales in January. In that case, we can perform a diagnostic analysis to determine what’s causing it.
-
Predictive Analysis
Based on past patterns and trends, we can perform predictive analytics to forecast what is likely to happen in the future. This is especially useful as it enables businesses to plan ahead. For example, streaming platforms such as Netflix and Youtube use their user views history, geographical location, search history, etc. to give movie/shows/videos recommendations to customers.
-
Prescriptive Analysis
Prescriptive analysis aims to understand what has happened, why it happened, and what should be done next based on the predicted outcomes. It enables us to see how individual conditions combination can impact the future/outcome. For example, when using map in our phone, it looks for all possible available routes (bus, car, train, etc.) and traffic conditions to suggest the best routes to get us from one place to another place and recommend.
We have reached the end of this blog, and hopefully, it has helped you to get more familiar with data and aware of the benefits we can gain from it by performing different types of data analytics depending on your needs.